
Computational Biology and Artificial Intelligence
Artificial Intelligence and Machine Learning at the Milner Therapeutics Institute
The aim of our Computational Biology and Artificial Intelligence team, led by Dr Namshik Han, is to create an atlas of disease mechanisms through the integration and interrogation of large multi-modal datasets, including multi-omics. The team are using bespoke machine learning methods to identify new signatures of disease and therapeutic targets, as well as graph-based algorithms to gain a deep understanding of the underlying causes of disease. The team have an established track record of collaboration with industry (e.g. GSK, AstraZeneca and Storm Therapeutics) and drug discovery partners (e.g. through a renewed partnership with LifeArc and also with Cancer Research Horizons/CRUK Cambridge Centre) through which they have developed and validated methodology with clear applications in drug discovery. The team works closely with researchers and clinicians in Cambridge and internationally (Europe, USA, and South Korea) who have unique patient datasets and disease models. It is this sharing of cross-sector expertise that ensures results are biologically interpretable and can inform go/no go decision making in drug discovery. The team’s approaches have been applied to target identification, validation, prioritisation and repositioning/repurposing, as well as biomarker discovery, and are applicable across many areas of healthcare including early detection and personalised medicine.
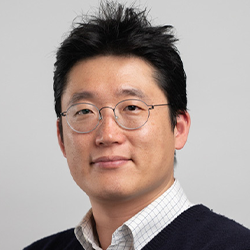
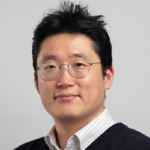
Discover our Research:
Group Members
Namshik Han (Head) Group Leader
|
Georgia Tsagkogeorga Senior Scientist
|
Gehad Youseff Postdoctoral Researcher
|
Holly Giles Postdoctoral Researcher
|
Nicholas Katritsis PhD Student |
Greta Baltusyte PhD Student
|
Sofia Radrizzani PhD Student
|
Seokjun Lee PhD Student
|
Fatima Baldo Associate Researcher
|
Jiwon Kim Undergraduate Student
|
Alessandra Cardinali Postdoctoral Scientist
|
Ragnor Comerford MPhil Student |
Xingze Xu PhD Student
|
Kyung Hun Chu Visiting Researcher
|
Zongtai Wu Masters Student
|
Nikhil Jain Masters Student |
Hyun Chang Lee PhD Student |
Jaesub Park Associate Researcher |
Alumni
Noah Collins
Woochang Hwang
Seungbeom Lee
Méabh MacMahon
Sophia Ramani
Ming Zeng
Soorin Yim
Lilly Wollman
Mark Wong
Rebecca Marrow
Louise Lecointre
Sanjay Rathee
Anika Liu
Lena Lampe
Ivan Zyuzin
Boris Vasilev
Winnie Lei
Selected Publications
Dennison et al.
Patient derived organoid biobank identifies epigenetic dysregulation of intestinal epithelial MHC-I as a novel mechanism in severe Crohn’s Disease.
Gut in print (2024)
MacMahon et al.
An in silico drug repurposing pipeline to identify drugs with the potential to inhibit SARS-CoV-2 replication.
Informatics in Medicine Unlocked 43, (2023)
Tsagkogeorga G et al
Predicting pathways associated with RNA methylation using machine learning.
Communications Biology 5, 868 (2022)
S Yim, W Hwang, N Han§, D Lee§.
Computational Discovery of Cancer Immunotherapy Targets by Intercellular CRISPR Screens.
Frontiers in Immunology (2022)
S Rathee*,§, M MacMahon*, A Liu, N Katritsis, G Youssef, W Hwang, L Wollman, N Han.
DILIC: An AI based classifier to search for Drug-Induced Liver Injury literature.
Frontiers in Genetics (2022)
A Liu, N Han, J Munoz-Muriedas, A Bender.
Deriving time-concordant event cascades from gene expression data: A case study for Drug-Induced Liver Injury (DILI).
PLOS Computational Biology (2022)
N Katritsis§, A Liu, G Youssef, S Rathee, M MacMahon, W Hwang, L Wollman, N Han.
Dialogi: Utilising NLP with chemical and disease similarities to drive the identification of Drug-Induced Liver Injury literature.
Frontiers in Genetics (2022)
W Hwang and N Han.
Identification of potential pan-coronavirus therapies using a computational drug repurposing platform.
Methods (2021)
W Hwang*, W Lei*, N Katritsis*, M MacMahon*, K Chapman and N Han.
Current and prospective computational approaches and challenges for developing COVID-19 vaccine.
Advanced Drug Delivery Reviews (2021)
Namshik Han*§, Woochang Hwang*, Konstantinos Tzelepis*, Patrick Schmerer*, Eliza Yankova, Méabh MacMahon, Winnie Lei, Nicholas M Katritsis, Anika Liu, Alison Schuldt, Rebecca Harris, Kathryn Chapman, Frank McCaughan, Friedemann Weber, Tony Kouzarides§.
Identification of SARS-CoV-2 induced pathways reveal drug repurposing strategies.
Science Advances (2021)
H Santos-Rosa*, G Millan-Zambran*, N Han*, L Pandolfini, T Leonardi, M Klimontova, S Nasiscionyte, L Pandolfini, K Tzelepis, Till Bartke and T Kouzarides.
Methylation of histone H3 at lysine-37 by Set1 and Set2 prevents spurious DNA replication.
Molecular Cell (2021)
P Amaral*, T Leonardi*, N Han*, E Vire, D Gascoigne, R Arias-Carrasco, M Büscher, L Pandolfini, Anda Zhang, S Pluchino, V Maracaja-Coutinho, H I. Nakaya, M Hemberg, RShiekhattar, A J. Enright and T Kouzarides.
Genomic positional conservation identifies topological anchor point RNAs linked to developmental loci.
Genome Biology (2018)
N Han§, H Noyes, A Brass§.
TIGERi: Modeling and visualizing the responses to perturbation of a transcription factor network.
BMC Bioinformatics (2017)
* Co-first author § Co-corresponding author
Case Study: LifeArc
Milner-LifeArc collaboration for novel disease signatures in oncology and infectious disease
The Milner Computational Research team, led by Namshik Han, have been collaborating closely with the medical research charity LifeArc over the last 3 years to develop and apply bioinformatics tools to identify novel therapeutic targets, biomarkers and drug repositioning opportunities.
By applying cutting-edge computational methods and analysis of multi-modal biomedical datasets, the team have identified new or better drug targets across a range of therapeutic areas. Key areas of research include the development and application of artificial intelligence, machine learning, statistical and mathematical approaches to pharmacogenomics and drug discovery.
Oncology research. LifeArc brings a rich oncology drug discovery programme to this collaboration. By developing methods to integrate LifeArc proprietary datasets with public cancer databases, the team have created a bespoke AI platform and computational pipeline to reveal novel disease signatures and then conducted agnostic pathway analysis to uncover new targets. Key outcomes from this work have included identification of new disease signatures for six cancer types in the LifeArc programme, and repositioning of an existing LifeArc target as a potential therapeutic strategy for an unanticipated cancer type.
COVID research. With the support of LifeArc, the group have also applied their bespoke AI methods and network analysis to uncover hidden pathways and reposition 200 already approved drugs against COVID-19. 20% of these drugs are currently in COVID-19 clinical trials, and the group went on to successfully validate two drugs in cellular assays (Proguanil and Sulfasalazine). These SARS-CoV-2 induced pathways define a resource for repurposing of drugs against COVID-19, either as monotherapies or in combination therapy.
“This collaboration has benefited enormously from the expertise of the LifeArc team in drug discovery. We see great potential to now build on the database resources and pipelines we have created together and apply them to reveal new disease signatures in oncology and other areas with high unmet medical need” says Namshik.
Dr David Pardoe, Head of Technology Development at LifeArc said:
“It is essential that we apply the power of massive and complex data to better understand fundamental biology and disease pathways. Doing this through the integration of different scientific disciplines represents just one of the ways we can collaboratively create real impact for patients”.
Case Study: Storm Therapeutics
The Milner Computational Research Group have been working with Storm Therapeutics for the past two years to apply machine learning techniques for prediction of novel human RNA methyltransferases.
Storm Therapeutics, co-founded by our Director Tony Kouzarides and Eric Miska (Gurdon Institute) is a Cambridge-based biotech company targeting RNA-modifying enzymes, including RNA methyltransferases, in the treatment of cancer.
RNA methyltransferases are known to functionally regulate RNAs, and have attracted increasing interest as potential drug targets given their newly identified role in cancer. There are many methylation sites known to exist on RNA, but enzymes have not been ascribed for many of them, suggesting that there are a number of yet to be identified RNA methyltransferases. Georgia Tsagkogeorga, a senior scientist at Storm, set out to identify new enzymes in this family with the Milner group, using the power of machine learning. By collecting and collating transcriptomic, proteomic, structural and protein-protein interaction data for human RMTs into a harmonized database, she built a large novel machine learning dataset. She has now applied four supervised machine learning models to this database, each of which produced novel predictions of RMTs. By consolidating the model outcomes to predict novel RMT genes, the team have now selected putative novel RMTs for further validation.
“This approach is demonstrating the strong potential of machine learning to interrogate large scale databases consisting of integrated multi-omics datasets and thereby make unanticipated predictions about this enigmatic enzyme family. We are excited about the potential of this partnership to identify new epigenetic regulators with therapeutic significance” says our Director Tony Kouzarides.